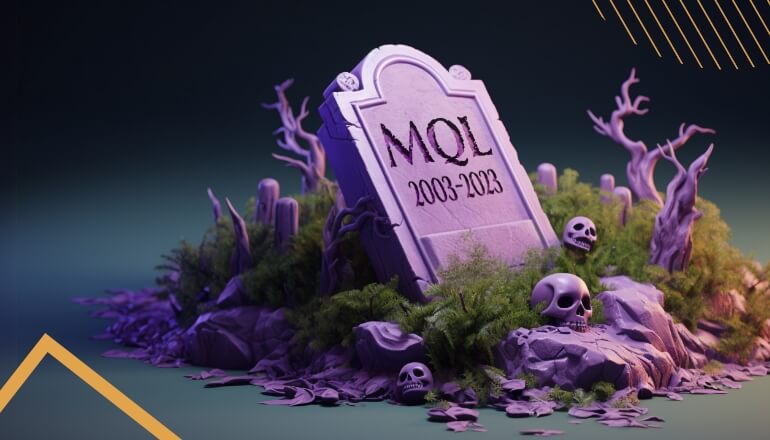
It's time for marketing and sales teams to change how we think about Marketing Qualified Leads (MQLs).
What is an MQL after all?
If you really think about it, an MQL is just a random point in time, right?
Hear me out...
We know that a new lead is a new person in our database and that a Sales Qualified Lead (SQL) is a lead that the sales team would approve of as qualified enough for the sales team to begin working. If that's true, then an MQL is just somewhere in between a lead and an SQL. An MQL can be someone who matches my Ideal Customer Profile (ICP) or someone who opened X number of emails.
But is that person really qualified?
During a recent HubSpot Hacks, we spoke with Kobe Stok, the co-founder and CEO of Forwrd, a Predictive AI app for qualification, forecasting, and retention, about the need for better lead qualification methods and to move away from MQLs and towards- AI Qualified Leads (AIQLs).
He explained that traditional lead scoring methods are broken. Still, by leveraging AI-driven analysis, businesses can unlock valuable insights from vast amounts of data and enhance their lead-scoring and conversion processes, leading to more closed deals. You can watch the entire episode here:
Sales and Marketing Alignment: Who is Really Qualified?
Moving from MQLs to AI-Qualified Leads (AI-QLs) requires addressing the all-to-common misalignment between marketing and sales teams when it comes to lead quality and quantity. The conversation usually goes something like this:
Sales: Hey, Marketing! I need more leads! I'll never hit my quarterly revenue goals if you don't send me more qualified leads!
Marketing: Hi, Sales, but I brought you these 1,000 leads. That should be more than enough to get you over the finish line for this quarter. Why aren't you working the leads?
Sales: I am working the leads, but these leads are no good! None of these leads fit our ICP, or if they do, they are not interested in taking a meeting with sales. Those that are willing to talk aren't closing any deals. You need to send me better-quality leads…
Marketing: Hmmm... No problem, Sales. Can you tell me a little more about what makes a good lead? The leads I'm sending you have the title and location that fits our ICP; they look like good leads to me...
Sales: Well, yes, but this one is from a company that is too small and that one had a use case that our solution doesn't solve for.
.... and they go around and around like that.
While marketing is generally focused on generating a high quantity of leads, sales may prioritize the quality of leads that have a higher likelihood of converting into customers.
This disconnect often leads to not only frustrated co-workers but also manual analysis of leads, assumptions based on limited information, and, ultimately, inefficient processes.
To bridge this gap, it is essential for marketing and sales teams to come together and agree on a unified set of goals and criteria for lead quality.
Dynamic Scoring for Lead Qualification
Once the two teams have agreed on their goals and set the criteria of what makes a qualified lead, it's then time to score the leads against that criteria. That is where the traditional approach to lead scoring falls short.
The rigid nature of assigning points based on linear rules fails to capture the dynamic context of each lead and the complexities of modern marketing strategies. As a result, we need a more comprehensive and adaptable approach to lead scoring.
To effectively evaluate leads' quality and potential, scoring methods must consider a wide range of attributes and behaviors. Simply relying on basic demographic information or engagement metrics is no longer sufficient. Instead, businesses must dig deeper and analyze various factors, including:
- Lead activity
- Website interactions
- Email engagement
- Pipeline stage
This is Where AI Comes In
By examining these (and potentially hundreds of other) diverse data points, companies can use AI models to get a much more thorough understanding of a lead's behavior, preferences, and, ultimately, their likelihood of conversion.
In fact, Stok told us that Forwrd's analysis found that lead scoring by traditional linear models had a 20% to 30% accuracy rate when predicting whether a lead would complete a business goal like becoming an SQL or closing a deal. In other words, you are better off flipping a coin about the lead's quality than using a traditional linear model.
Traditional linear lead-scoring models
have a 20% to 30% accuracy rate. 😳
The Power of AI-Fueled Lead Scoring
By comparison, AI-qualified leads have a 75% to 90% accuracy rate when predicting whether a lead would complete a business goal like becoming an SQL or closing a deal.
AI-Qualified leads have a 75% to 90% accuracy rate.
Al tools are able to predict which leads are more likely to convert into new business because they are able to quickly analyze vast amounts of historical data and gain deeper insights into the various life cycles of leads.
By analyzing data such as time spent on a specific page or clicks on a specific link in an email, the AI tool can assign a more accurate score to each lead, reflecting their potential to become a sales-qualified lead or closed deal.
Moreover, AI models give companies the ability to adapt and evolve their lead scoring over time. Customer preferences and business strategies are constantly changing, and lead-scoring models should reflect these shifts. By continuously monitoring and analyzing data, AI tools can identify patterns and trends to update scoring criteria.
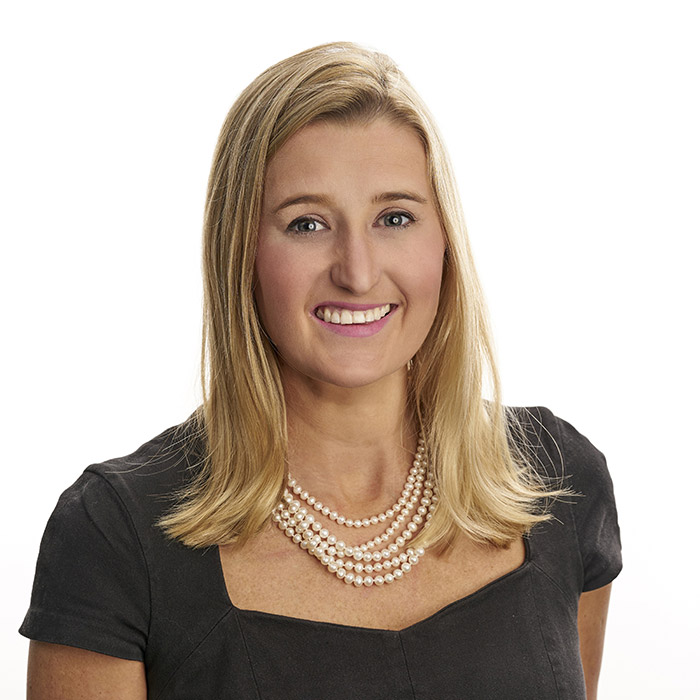